言語モデリングへの普及が2025年にテキストを改善する3つの方法
AI で生成されたテキストが、時間の経過とともにより一貫性があり意味のあるものになったことにお気づきでしょう。 言語モデリングへの普及 AI がテキストを作成する方法を改良することで、この変革を推進しています。古いモデルとは異なり、拡散モデルは反復的な改良によってパフォーマンスを向上させます。たとえば、拡散ベースのアプローチであるDEEMでは、RobustVQAなどのタスクの結果が 4%、MMVP が 6.5%、POPE が 12.8% 向上します。これらの進歩により、AI が生成するテキストはより正確で文脈に応じたものになります。ストーリーの作成であれ、複雑な質問への回答であれ、拡散モデルは2025年にテキスト生成の新しい基準を打ち立てます。
言語モデリングにおける拡散モデルとは
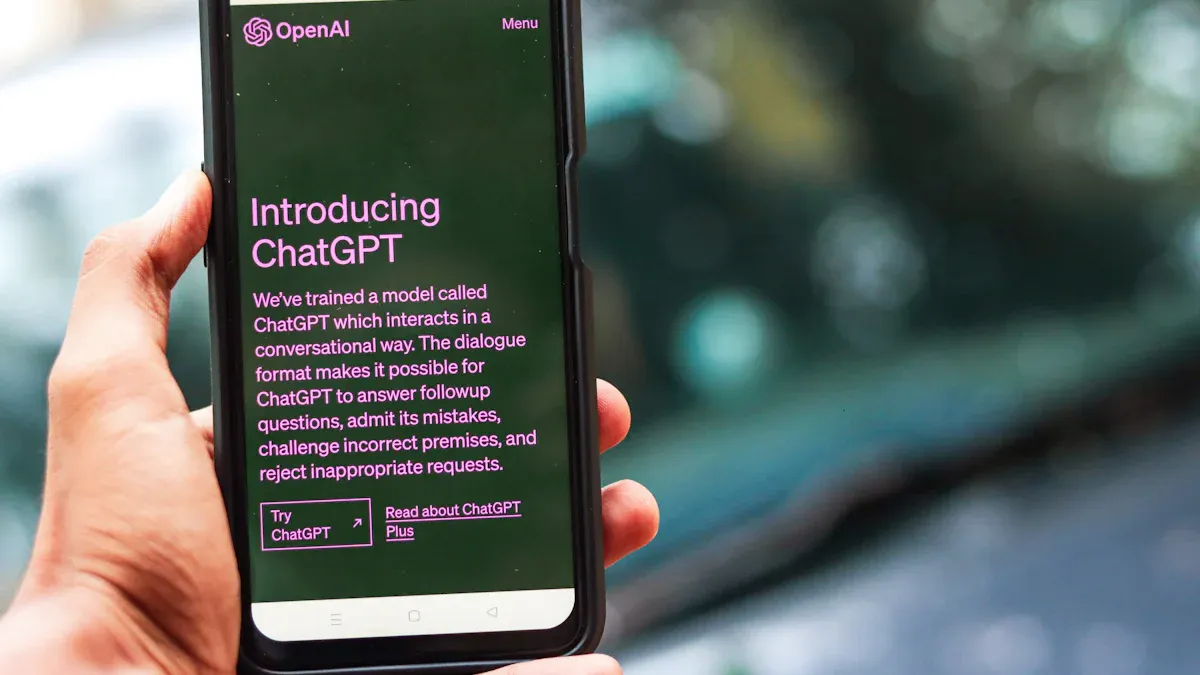
拡散モデルの基本
反復的改良による拡散モデルの仕組みの概要
拡散モデルは、テキストを段階的に調整して作成します。このプロセスは、大まかな下書きを明確で一貫性のあるものになるまで磨き上げるものと考えることができます。これらのモデルはまずランダムノイズから始め、徐々に意味のあるテキストに変換します。各ステップで品質が向上し、最終出力が意図したコンテキストと一致するようになります。この反復的なアプローチは、繰り返しの多いフレーズや無関係な詳細などの一般的な問題を回避するのに役立ちます。
ディフュージョンモデルは大理石のブロックを形作る彫刻家のように振る舞い、その中に隠された傑作を明らかにします。
この改良プロセスにより、拡散モデルは、シングルパスでテキストを生成することが多い従来の方法とは一線を画しています。タスクを小さなステップに分割することで、拡散モデルはより高い精度とより優れたコヒーレンスを実現します。
画像生成からテキスト生成への移行
拡散モデルは画像生成で初めて人気を博しました。ディフュージョンモデルは、ピクセルデータを改良して見事なビジュアルを生み出しました。研究者たちはすぐに、これらのモデルがテキスト生成にも変革をもたらす可能性があることに気付きました。彼らはピクセルを扱う代わりに、単語や文章を処理するようにプロセスを調整しました。
この移行がどのように機能するのか不思議に思うかもしれません。テキスト生成では、拡散モデルは言語を一連のデータポイントとして扱います。これらのポイントを段階的に改良して、テキストが自然に流れ、意味のあるものになるようにします。このアプローチは、視覚的創造性と言語的創造性のギャップを埋め、AI 主導のストーリーテリングとコミュニケーションの新たな可能性を開きます。
潜在言語拡散モデルの役割
テキスト生成に潜在空間がどのように使用されるかの説明
潜在言語拡散モデルは、改良プロセスをさらに一歩進めます。これらはテキストを数学的に表現した「潜在空間」で動作します。これらのモデルは、単語を直接扱うのではなく、テキストを連続した空間にマッピングします。これにより、パターンや関係を分析しやすくなります。
潜在空間を、アイデアと意味がシームレスに融合するキャンバスとして想像してみてください。この空間で作業することで、拡散モデルはより自然で直感的に感じられるテキストを生成できます。この方法により、モデルは微妙なニュアンスや複雑なアイデアを捉えることができるため、ストーリーテリングや詳細な質問への回答などのタスクに最適です。
不連続テキストを連続空間にマッピングするメリット
不連続テキストを連続空間にマッピングすることには、いくつかの利点があります。1 つ目は、厳密な単語単位の処理によって発生するエラーのリスクが軽減されることです。2 つ目は、モデルがコンテキストをよりよく理解できるようになり、生成されたテキストが確実に期待どおりになることです。
たとえば、潜在言語拡散モデルにストーリーを書いてもらう場合、単語をつなぎ合わせるだけではありません。登場人物、出来事、感情の関係を考慮して、本物らしさを感じさせる物語を作り出します。このような複雑さへの対応能力により、潜在言語拡散モデルは AI 主導のテキスト生成におけるゲームチェンジャーとなります。
潜在言語拡散モデルは、創造性と精度を融合させることで、言語モデリングでAIが達成できることを再定義します。
なぜ拡散モデルがテキスト生成で注目されているのか?
従来のモデルの限界への対処
反復的で一貫性のないテキスト生成の克服
従来のテキスト生成モデルでは、繰り返しの多いフレーズや一貫性のない出力に苦労することがよくあります。拡散モデルは、テキストを段階的に改良することでこれらの問題を解決し、反復するたびに品質が向上するようにします。このプロセスにより、モデルは文脈と意味に焦点を当て、自然で魅力的なテキストを作成できます。
たとえば、DesignDiffusion フレームワークでは、高度な手法を使用してテキスト生成を強化しています。文字レベルの分解を使用して各要素を正確にレンダリングします。文字ローカリゼーション損失関数を使うと、テキストが目的の領域に揃えられるため、精度がさらに向上します。これらの戦略は、困難なシナリオでも拡散モデルが一貫性のある高品質なテキストを生成するのに役立ちます。
これらの制限に対処することで、拡散モデルはAIによるテキストの生成方法を再定義し、ストーリーテリング、会話作成、コンテンツマーケティングなどのタスクにおける信頼性を高めます。
長文で複雑なコンテンツの処理が改善されました
長い形式のコンテンツは従来のモデルを圧倒することが多く、アイデアが断片化され、一貫性のない物語になってしまいます。拡散モデルは、潜在的な空間の中でアイデア間の関係を分析し、反復的に改良していくことで、この分野で優れています。このアプローチにより、生成されたテキストは拡張フォーマット間で一貫性と深みを維持できます。
セルフプレイ・ダイレクト・プリファレンス・オプティマイゼーション(SP-DPO)は、モデルのアウトプットを人間の好みに合わせることで、この機能をさらに強化します。広範囲にわたる実験により、拡散モデルは複雑で微妙なテキストを生成する点で古い方法よりも優れていることが示されています。詳細なレポートが必要な場合でも、説得力のあるストーリーが必要な場合でも、これらのモデルは品質と関連性の高い基準を満たす結果をもたらします。
2025年の拡散モデルの進化
クリエイティブでコンテキスト認識型の AI 生成テキストに対する需要の高まり
2025年には、クリエイティブでコンテキストを意識したテキスト生成の需要が新たな高みに達しました。マーケティング、広告、E コマースなどの業界では、視聴者の心に響く魅力的なコンテンツを制作するために AI が活用されています。拡散モデルは、他に類を見ない創造性と正確性を提供することで際立っています。
テキストから画像への生成市場はこの傾向を浮き彫りにしており、2033年までに数十億ドル規模の評価額が見込まれています。AI とディープラーニングの進歩がこの成長を後押しし、拡散モデルがもたらす変革的な影響が明らかになっています。Stable Diffusion のようなオープンソースツールはアクセスを民主化し、ユーザーがテキスト生成における革新的なアプリケーションを模索できるようにします。
このような採用の増加は、パーソナライズされたマーケティングキャンペーンの作成から教材の作成まで、さまざまなタスクを処理できるAIシステムの必要性を反映しています。拡散モデルは、創造性とコンテキスト認識を組み合わせることでこれらの要求に応え、AI 主導のテキスト生成の新しいベンチマークを打ち立てます。
マーケティング、教育、カスタマーサービスなどの業界でのアプリケーション
拡散モデルは、さまざまな業界で不可欠になっています。マーケティングでは、注目を集める説得力のある広告コピーや商品説明を生成します。教育分野では、学生のニーズに合わせたインタラクティブな学習教材を作成します。カスタマーサービスには、正確で共感的な応答を提供する AI 主導のチャットボットが役立ちます。
これらのアプリケーションは、拡散モデルの多様性を際立たせています。反復プロセスを通じてテキストを絞り込むことで、特定の目標や文脈に沿ったアウトプットが得られます。顧客エンゲージメントの強化や教育成果の向上など、目的がどのようなものであっても、拡散モデルはニーズに合ったソリューションを提供します。
言語モデリングへの普及が2025年にテキストを改善する3つの方法
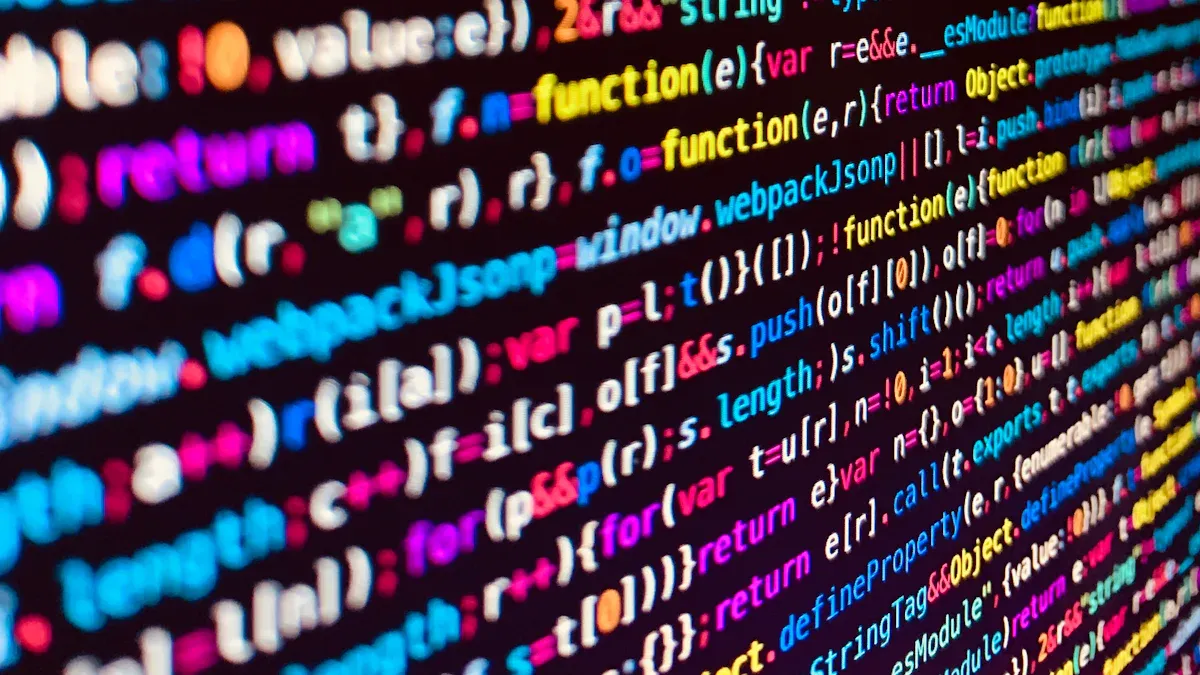
1。テキストの一貫性とコンテキスト認識の強化
拡散モデルがコンテキストとより一致したテキストを生成する方法
言語モデリングへの普及により、AIがテキストを理解して生成する方法が再定義されました。これらのモデルは、アウトプットを入力のコンテキストに合わせるのに優れています。テキストを繰り返し調整することで、すべての単語や文がより広い物語に自然に収まるようにします。このプロセスにより、古いモデルで発生していたような、ばらばらになったり、無関係な応答がなくなります。
たとえば、拡散モデルにストーリーを書いてもらう場合、個々の文章だけに焦点を当てるわけではありません。登場人物、設定、出来事の関係性を考慮して、まとまりのある魅力的な物語を作り出します。この能力は、潜在空間に潜むテキストを分析して調整するモデルの能力に由来し、入力の根底にある構造と意味を捉えます。
このアプローチの有効性は、パフォーマンス指標から明らかです。拡散モデルは、一貫性、関連性、忠実性において目覚ましいスコアを達成しています。
ストーリーテリングとダイアログ生成の改善例
小説の台詞を作っているところを想像してみてください。従来のモデルでは一般的な線や反復的な線が生成される場合がありますが、拡散モデルではさらにその傾向が強くなります。それぞれのキャラクターの声や個性が輝き渡り、本物らしく感じられる会話を生み出します。
ストーリーテリングでは、これらのモデルはプロットの一貫性を維持するのに優れています。たとえば、ストーリーにミステリーが含まれている場合、モデルは手がかりやひねりが物語の流れと一致していることを確認します。この機能により、拡散モデルはクリエイティブライティングやその他のテキスト生成作業に非常に役立ちます。
拡散モデルを使用すると、テキストが意味をなすだけでなく、聴衆の共感を呼ぶことを信頼できます。
2。テキスト出力における創造性と多様性の向上
ユニークで想像力豊かなテキストを作成する能力
拡散モデルは、テキスト生成に新たなレベルの創造性をもたらします。潜在空間で作業することで、幅広い可能性を探求し、ユニークで想像力に富んだアウトプットを生み出します。このアプローチにより、詩を書いたり、マーケティングコピーを作成したり、アイデアをブレインストーミングしたりする場合でも、目立つテキストを生成できます。
たとえば、拡散モデルは、従来のモデルでは見過ごされがちな鮮明な説明やメタファーを作成できます。また、フォーマルなトーンでも遊び心のあるトーンでも、好みに合わせてスタイルを調整できます。この柔軟性により、出力のスタイルや内容を正確に制御する必要があるような、制御されたテキスト生成に最適です。
クリエイティブライティング、マーケティング、エンターテイメントへの応用
クリエイティブライティングでは、拡散モデルは新鮮なアイデアや視点を提供することで、ライターズ・ブロックを打破するのに役立ちます。マーケティングでは、注目を集め、エンゲージメントを促進する説得力のある広告コピーを生成します。エンターテインメント業界は、視聴者の共感を呼ぶ脚本、会話、さらには歌詞さえも作成できるというメリットがあります。
これらのアプリケーションは、拡散モデルの多様性を示しています。創造性と精度を組み合わせることで、ニーズを満たすだけでなく、期待を超えるテキストを作成できます。
3。あいまいさやニュアンスの処理の改善
拡散モデルがどのように微妙な言語を解釈して生成するか
あいまいさや微妙なニュアンスは AI にとって課題となることがよくありますが、拡散モデルなら簡単に処理できます。これらのモデルは、潜在空間におけるユーザーの入力を分析し、微妙な意味や関係を捉えます。このプロセスにより、人間の言語の複雑さを反映したテキストを生成できます。
たとえば、拡散モデルに概念を説明するように依頼しても、単純な答えが得られるだけではありません。詳細な説明を求めているのか、簡潔な要約を求めているのかに関わらず、コンテキストを考慮し、ニーズに合わせて対応を調整します。このような適応能力により、拡散モデルは言語を深く理解する必要があるタスクで特に効果的です。
会話型AIとカスタマーサポートにおけるより良い対応の例
会話型 AI では、拡散モデルは自然で共感できるような応答を生成するのに優れています。たとえば、カスタマーサポートのチャットボットとやり取りする場合、モデルは、友好的な口調を保ちながら、回答がお客様の懸念事項に対処することを保証します。
カスタマーサポートでは、これらのモデルは複雑なクエリを簡単に処理できます。質問のニュアンスを分析し、状況に応じた正確な回答を提供します。この機能により誤解が減り、全体的なユーザーエクスペリエンスが向上します。
あいまいさとニュアンスをマスターすることで、拡散モデルはAI主導型コミュニケーションの新しい基準を打ち立てます。
推奨ツール:AI 主導のコンテンツ作成のための PageOn.ai
PageOn.ai の概要
AI 検索および仮想プレゼンテーションツールとしての PageOn.ai の紹介
PageOn.ai は、コンテンツの作成とプレゼンテーションを簡素化するために設計された最先端のツールです。AI 主導の検索機能と仮想プレゼンテーション機能を組み合わせることで、専門家にとっても学生にとっても不可欠なリソースとなっています。レポート、マーケティングピッチ、教育用プレゼンテーションのいずれを作成する必要がある場合でも、PageOn.ai はアイデアを洗練された視覚的に魅力的なアウトプットに変えるのに役立ちます。
AI 主導の検索、コンテンツ生成、視覚化をどのように組み合わせているか
このツールは、AI検索、コンテンツ生成、視覚化を1つのシームレスなプラットフォームに統合します。トピックやアイデアを入力すると、PageOn.ai がニーズに合わせた構造化されたコンテンツを生成します。また、グラフ、画像、レイアウトなどの視覚的要素も提供されるため、情報量が多く、視覚的にも魅力的なプレゼンテーションを作成できます。これらの機能を組み合わせることで、PageOn.ai は時間と労力を節約し、プロ並みの品質を実現できます。
PageOn.ai の主な機能
Vibe Creation: 音声またはテキストを使用して構造化されたビジュアルコンテンツを生成します
Vibe Creation機能を使用すると、アイデアを話したり入力したりするだけでコンテンツを作成できます。このツールは入力内容を明確な構造に整理し、理解を深めるために視覚的な要素を追加します。この機能は、ブレインストーミングセッションや、プロジェクトの概要をすばやく説明する必要がある場合に最適です。
AI ブロック:コンテンツブロックをドラッグアンドドロップしてシームレスにカスタマイズ
PageOn.ai には AI ブロックが用意されており、コンテンツを簡単にカスタマイズできます。あらかじめデザインされたブロックをドラッグアンドドロップしてプレゼンテーションを作成できます。この機能により柔軟性が確保され、レイアウト、テキスト、ビジュアルを特定の要件に合わせて調整できます。
ディープサーチ:ビジュアル、データ、チャートを簡単に統合
ディープサーチ機能を使用すると、関連するビジュアル、データ、チャートを検索してコンテンツに統合できます。この機能により、プレゼンテーションは参考になるだけでなく、正確なデータにも裏付けられます。プロフェッショナルな要素を追加するプロセスを簡素化し、作品を際立たせることができます。
エージェント能力:アイデアを洗練されたプレゼンテーションに変える
PageOn.ai のエージェント機能は、未加工のアイデアを、完全に洗練されたプレゼンテーションに変換します。このツールは、入力内容を分析して改善点を提案し、最終的なアウトプットが目標に沿っていることを確認します。この機能は、厳しい締め切りの中でインパクトのあるプレゼンテーションを作成する場合に特に便利です。
PageOn.ai の使用に関するステップバイステップガイド
PageOn.ai のウェブサイトにアクセスして、トピックやアイデアを入力してください
まず、PageOn.ai プラットフォームにアクセスしてください。検索バーにトピックやアイデアを入力して、コンテンツ作成プロセスを開始します。
参考ファイル (PDF、CSV、マークダウンなど) のアップロード
参考資料がある場合は、それらをアップロードして追加のコンテキストを提供してください。ツールはこれらのファイルを使用して、より正確で関連性の高いコンテンツを生成します。
AI が生成したアウトラインを確認してテンプレートを選択
ツールがアウトラインを生成したら、それを確認してニーズに合っていることを確認します。プレゼンテーションのトーンやスタイルに合わせて、さまざまなテンプレートから選択してください。
AI Chat を使用してテキスト、ビジュアル、グラフのコンテンツをカスタマイズ
AI チャット機能を使用してコンテンツを絞り込みます。テキスト、ビジュアル、チャートを追加または編集して、魅力的で有益なプレゼンテーションを作成できます。
プレゼンテーションをクラウドに保存するか、PDF としてダウンロードしてください
プレゼンテーションが完成したら、簡単にアクセスできるようにクラウドに保存するか、PDF としてダウンロードします。この柔軟性により、聴衆に合った形式で作品を共有できます。
PageOn.ai を使用すると、最小限の労力でプロ品質のコンテンツを作成できます。その機能によりプロセスが合理化され、インパクトのあるメッセージの配信に集中できます。
PageOn.ai が目立つ理由
アイデアをインパクトのあるビジュアルに変えるプロセスを簡素化します
PageOn.ai を使うと、プロ並みのプレゼンテーションを簡単に作成できます。アイデアだけから始めることも、大まかなアウトラインから始めることもできます。プラットフォームはそれを洗練されたビジュアルの傑作に変えます。AI 主導の検索ツールとプレゼンテーションツールが連携することで、時間と労力を節約できます。PageOn.ai を使用すれば、コンテンツの調査や整理に何時間も費やすことなく、関連情報をすばやく見つけることができます。その後、ツールはこのデータを明確で説得力のあるストーリーに整理し、メッセージをよりインパクトのあるものにします。
直感的な編集ツールにより、プレゼンテーションを簡単にカスタマイズできます。高度なデザインスキルがなくても、レイアウトの調整、ビジュアルの追加、テキストの調整を行うことができます。このシンプルさにより、経験に関係なく、誰でも印象に残るプレゼンテーションを作成できます。
PageOn.ai を使用すると、プラットフォームが設計と構造という面倒な作業を処理している間、アイデアに集中できます。
リアルタイムのコラボレーションとデータ視覚化ツールを提供
PageOn.ai を使用するとコラボレーションがシームレスになります。このプラットフォームはリアルタイムのチームワークをサポートしているため、あなたと同僚が同じプロジェクトに同時に取り組むことができます。この機能により、メールをやり取りしたり、バージョン管理の問題が発生したりする必要がなくなります。アイデアの共有、編集、プレゼンテーションの完成を、すべて同じインターフェイス内でまとめて行うことができます。
データ視覚化ツールはプレゼンテーションをさらに強化します。PageOn.ai は、チャート、グラフ、その他の視覚要素を簡単に統合します。これらの機能により、複雑な情報をわかりやすく表示できます。トレンドを説明する場合でも、指標を比較する場合でも、結果を紹介する場合でも、プラットフォームはデータがプロフェッショナルで魅力的に見えるようにします。
リアルタイムのコラボレーションとダイナミックなビジュアルにより、PageOn.ai はチームにとっても個人にとっても強力なツールとなっています。
AI画像生成を統合してパーソナライズされたプレゼンテーションを実現
記憶に残るプレゼンテーションを作成するには、パーソナライズが重要です。PageOn.ai はこの点で優れています。このプラットフォームは AI 画像生成を使用して、コンテンツに合わせたビジュアルを作成します。トピックにぴったり合ったカスタム画像を生成して、プレゼンテーションを目立たせることができます。
この機能は、ストックライブラリにはないユニークなビジュアルが必要な場合に特に便利です。たとえば、未来的なコンセプトやニッチなアイデアを提示する場合、AI はビジョンに合った画像を作成できます。これらのパーソナライズされたビジュアルはクリエイティブなタッチを加え、プレゼンテーションをより魅力的でインパクトのあるものにします。
PageOn.ai は、インテリジェントなデザインとカスタマイズを組み合わせることで、聴衆の心に響くプレゼンテーションを実現するのに役立ちます。
言語モデリングにおける拡散モデルの課題と限界
計算の複雑さとリソース要件
従来のモデルと比較して高い計算コスト
拡散モデルは大量の計算リソースを必要とし、多くのユーザーがアクセスしにくくなります。このようなモデルのトレーニングには、多くの場合、数百の GPU 日が必要であり、V100 や A100 GPU のようなハイエンドなセットアップが不可欠です。たとえば、A100 GPU では 50,000 個のサンプルを生成するのに最大 5 日かかることがあります。このような大量のリソース消費は、小規模な組織や独立した研究者にとって障壁となります。
拡散モデルからのサンプリングでは、高品質なアウトプットを生成するために、多くの場合25~50ステップの複数の評価が必要になります。漸進的蒸留法は推論を高速化できますが、追加のトレーニングが必要になり、計算負荷がさらに増大します。これらの課題は、拡散モデルをより効率的かつ利用しやすいものにするための最適化手法の必要性を浮き彫りにしています。
高度なハードウェアと最適化技術の必要性
拡散モデルの複雑さは、高度な数学的手法に依存していることに起因しています。出力の微調整には、モンテカルロシミュレーションや有限差分法などの手法がよく使用されます。これらの方法は効果的ですが、かなりの処理能力とメモリを必要とします。
これらの課題に対処するための取り組みには、重みの精度を低下させるモデル量子化や、効率を向上させるアクティベーションが含まれます。量子化対応トレーニング (QAT) やトレーニング後量子化 (PTQ) などのアプローチは、拡散モデルの高速化とメモリ効率の向上を目的としています。ただし、これらの手法の実装には専門知識と専用のハードウェアが必要であり、普及には限界があります。
倫理上の懸念と誤用
誤解を招くコンテンツや有害なコンテンツを生成するリスク
ディフュージョンモデルには計り知れない創造力がありますが、この力にはリスクが伴います。AI が生成したコンテンツは、誤った情報を広めたり、政治的怒りを扇動したりするために操作される可能性があります。たとえば、偽の画像や動画は、メディアへの信頼を損なうために世界中で使用されています。例としては、イスラエル・ハマスの紛争中の誤解を招くような映像や、ウクライナ大統領ゼレンスキーが降伏した偽造ビデオなどがあります。
モデルの崩壊も懸念事項です。汚染されたデータや偏ったデータで拡散モデルをトレーニングすると、不正確さが助長され、現実が歪んでしまいます。この問題は、AI の倫理的利用とデータインテグリティの維持の重要性について疑問を投げかけています。ニューロライトをめぐる議論では、認知の自由とプライバシーを保護し、人工知能が認識を操作したり個人の権利を侵害したりしないようにする必要性が強調されています。
責任ある AI の開発と展開の重要性
これらのリスクを軽減するために、開発者は倫理的ガイドラインと透明性を優先する必要があります。責任ある AI プラクティスには、偏見を防ぐための厳格なテストや AI 生成コンテンツの明確なラベル付けが含まれます。政策立案者や研究者は、AIが害ではなく進歩のツールとして役立つことを保証し、誤用を防ぐフレームワークを提唱しています。
拡散モデルの限界についてユーザーを教育することも同様に重要です。悪用の可能性を理解することで、AI が生成したコンテンツに批判的にアプローチし、情報に基づいた意思決定を行うことができます。この認識は、AI テクノロジーに対する説明責任と信頼の文化を育みます。
統合の課題
拡散モデルを既存のワークフローに統合することの難しさ
拡散モデルを採用するには、多くの場合、既存のシステムに大幅な変更を加える必要があります。これらのモデルは潜在空間で機能し、従来の方法とは異なる反復的な改良に頼っています。それらをワークフローに統合するには、技術的な専門知識とアーキテクチャに関する深い理解が必要です。
組織は、拡散モデルをサポートするようにインフラストラクチャを適応させる際に課題に直面しています。高度なハードウェアと専用のソフトウェアが必要なため、このプロセスは複雑になり、技術的なバックグラウンドを持たないチームがこれらのモデルを効果的に実装することが難しくなります。
ユーザーフレンドリーなツールとプラットフォームの必要性
統合の障壁を克服するには、開発者は拡散モデルの使用を簡素化するツールを作成する必要があります。直感的なインターフェースと自動化されたプロセスを備えたプラットフォームは、技術的な複雑さと実用的なアプリケーションの間のギャップを埋めるのに役立ちます。たとえば、ドラッグアンドドロップ機能を提供するツールや、あらかじめ作成されたテンプレートを使用すると、拡散モデルをさまざまなタスクに簡単に組み込むことができます。
アクセシビリティに重点を置くことで、開発者は拡散モデルがより多くのユーザーに届くようにすることができます。このアプローチは、使いやすさを向上させるだけでなく、マーケティング、教育、カスタマーサービスなどの業界全体でこれらのモデルの可能性を最大限に引き出すことにもなります。
拡散モデルは、一貫性、創造性、ニュアンスを向上させることで、AIがテキストを生成する方法を変えています。これらのモデルはアウトプットを段階的に改良し、従来のアプローチよりも効果的なものにしています。たとえば、テキスト生成タスクでは、1.1B マスク拡散モデル (MDM) が 13B Llama-2 や 175B GPT-3 などの大規模モデルを上回っています。これはその効率性と可能性を示しています。
高い計算コストや倫理的な懸念などの課題は存在しますが、利点は欠点を上回ります。PageOn.ai のようなツールを使用すると、AI を活用して視覚的に魅力的でアクセスしやすいコンテンツを作成できます。これらの進歩により、複雑なアイデアをインパクトのあるメッセージに簡単に変換できます。